The Unlikely Allies: A Vision For AI-Assisted Privacy Operations
There is an immense opportunity for AI to address data privacy challenges and have a massive impact on privacy operations.
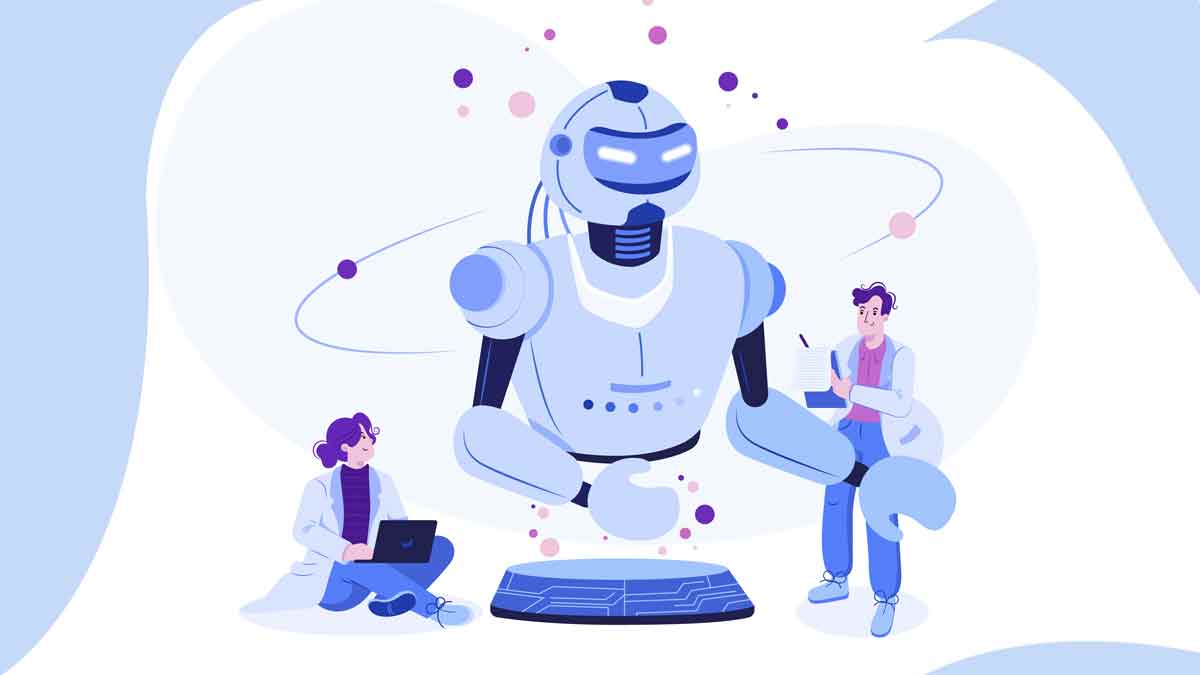
AI Wars Have Arrived
A New York Times reporter was unsettled by it. Italy's data protection agency banned it. Bill Gates calls it a revolutionary technology that will change the world but Elon Musk considers it one of the biggest risks for the future of civilization.
AI governance is getting a lot of renewed attention since the recent popularity of advanced AI tools, particularly ChatGPT. Tech luminaries and renowned scientists have asked for a temporary pause on their further development. While ethical and privacy concerns no doubt exist, the clarion calls for avoiding the use of AI may not be heeded any more than the declarations of "code red" to continue to push the boundaries of this technology.
Put simply, the AI wars are here to stay, and fight we must against all odds to balance the opportunities and challenges. An Italian minister who called his country's ban on ChatGPT excessive said that "every technological revolution brings great changes, risks and opportunities," while Bill Gates noted that "we should try to balance fears about the downsides of AI with its ability to improve people’s lives."
This is true for AI's impact on data privacy as well.
Privacy Challenges = AI Opportunities
Just as much as AI opportunities create data privacy challenges, there exist data privacy challenges that create opportunities for AI to address them. It is within this context that this post lays out a vision for AI-assisted data privacy operations.
While an ideal organization may have the right combination of tools and team members with sufficient budget, many organizations in practice do not have sufficient resources, and either lack tools or have tools that are under-utilized due to lack of expertise or capability.
These are the kind of challenges that AI can help overcome. Imagine how much productivity boost it will be to have AI assist with privacy operations that not only makes up for the resources and expertise but also helps improve compliance ROI.
Let's discuss with a few examples how we can get there.
Primary Use Cases
The chart below captures a few primary use cases where AI can assist with data privacy operations. These represent foundational requirements under most data privacy laws and putting them in place would help an organization achieve a basic level of data privacy maturity.
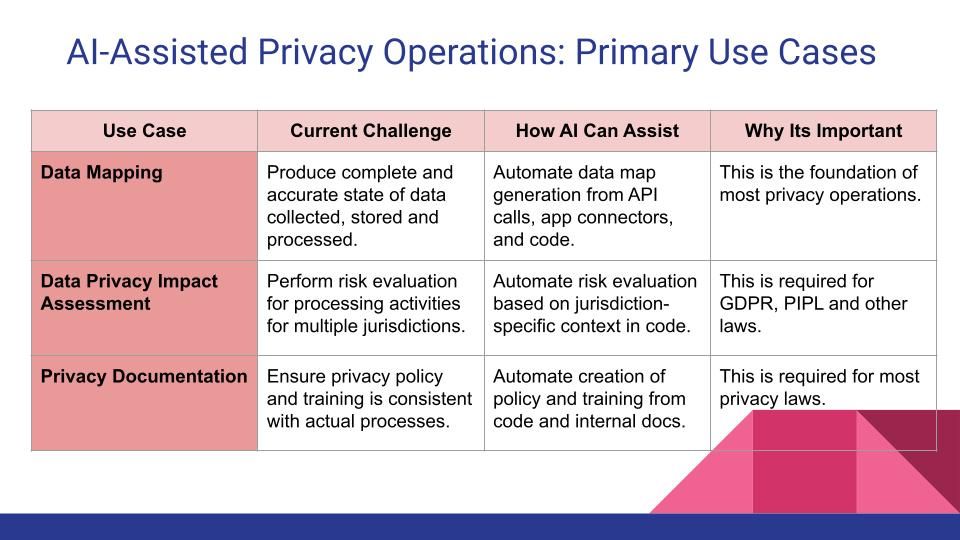
Data Mapping: Having a data map is the foundation for most privacy operations, as it tells you what data you have, where and how its stored, what you use it for, and who do you share it with. The current challenge faced by organizations is to be able to produce a complete and accurate data map. This is primary due to data in unknown locations and/or from unknown sources and/or or for unknown activities. AI can help address this challenge by automating the processing of discovery based on the actual state of the system that is represented by the apps connected to it, the API calls, and the code.
Data Privacy Impact Assessment: The ability to perform a privacy risk assessment is central to most data privacy laws across multiple jurisdictions. However, it can be labor intensive and significantly burden the development processes of the organization. AI can help automate the process by learning from information in the code that can help evaluate the risk of a processing activity. This not only makes it more efficient but also more accurate and consistent, and allows it to be produced in a standardized manner for regulators and auditors.
Privacy Documentation: Having a policy and training that is consistent with the practices of the organization with regards to data privacy is a principle requirement in most privacy laws. However, due to resource constraints, organizations may rely on cookie cutter policies and trainings that are not always aligned with their actual practices. AI can be used to automate the process of creating policy and training materials directly from code or internal product documents, and therefore helps keep them consistent with the actual processes of the organization.
Advanced Use Cases
The chart below captures a few advanced use cases where AI can assist data privacy operations. Implementing them would allow an organization to achieve an advanced level of data privacy maturity, and improve the overall efficiency of its privacy operations.
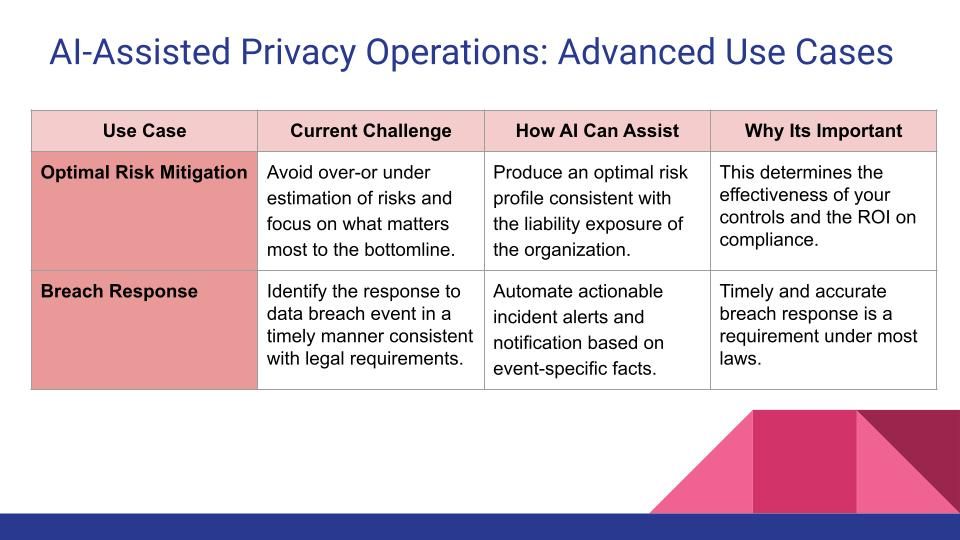
Optimal Risk Mitigation: Companies often over- or under-estimate their risk of non-compliance. From a business perspective, its important to focus on what matters most to the bottomline. We can optimize for it by “right-sizing” the risk profile- the process of establishing technical and legal controls consistent with the liability exposure of the organization.
Advanced AI models can be safely trained on industry-specific context data with proper constraints. With sufficient amount of training in data privacy context, an AI model can be used to output an optimal risk profile for that industry. Such context data will comprise the likely indicators of exposure and/or compromise (e.g. unencrypted, publicly accessible S3 buckets that contain sensitive data), the associated legal liability (e.g., penalty per exposed record), along with the controls most commonly associated with mitigating that risk (e.g., encryption). It will also take into account compensating controls (such as breach response retainers and cyber insurance) to mitigate the residual risk of cyber loss.
If the model has already been trained to perform a Data Privacy Impact Assessment (DPIA) to highlight the prioritized risks, the process of right-sizing the risk profile can build on top of that with the help of the additional context data. This vision appears within reach, as products such as DroidGPT in the security domain are already emerging that combine the power of AI models with domain-specific risk insights to perform risk prioritization.
Breach Response: Advanced AI models can be trained to respond to specific scenarios, such as a data breach event, with actionable data privacy guidance based on given facts. This would be similar to how Microsoft is training its Security Co-pilot to deliver data security guidance that accelerates incident investigation and response.
Below is how ChatGPT responded to a prompt regarding a company's obligations and liabilities for a data breach scenario involving encrypted data of CA residents:
Based on these facts, ChatGPT did the correct legal analysis of the data breach scenario. It pointed out the notification obligation and liability under the law, noted that encryption may provide exemption (depending on its strength), and mentioned reasonable security as a factor in avoiding liability.
This can go a step further, and the whole process can be automated along the lines of how Zapier is already supporting integration with Open AI. Its reasonable to envision a mechanism (such as a webhook) where an event (such as detecting a data exposure) triggers the prompt with specific facts (such as jurisdiction of affected users, encryption status of affected data) to the model which produces an actionable output (such as requiring breach notification).
The response can then be used to alert the incident commander (IC) of what actions to take next. In some cases the actions may be recognized from the response and either fully automated or available for the IC to perform with one click (e.g. issue an auto-drafted notification letter).
Conclusion
In conclusion, there is an immense opportunity for AI to address data privacy challenges and have a massive impact on privacy operations. Generative. Pre-Trained. Transformative. That’s how your privacy infrastructure should be.
We are not there today, but can get there with sufficient amount of fine-tuning of advanced AI models. There is already a lot of momentum in doing that for solving domain-specific problems. In order to avoid privacy concerns, organizations can build ChatGPT-like clones in their own environment, or build custom models from scratch for their target domain. All of this contributes toward making AI-assisted privacy operations a reality.
Connect with us
If you would like to reach out for guidance or provide other input, please do not hesitate to contact us.