Responsible Innovation: A Checklist of AI Governance Requirements
In order to realize the potential of responsible AI innovation, organizations must be able to translate AI governance requirements into technical controls.
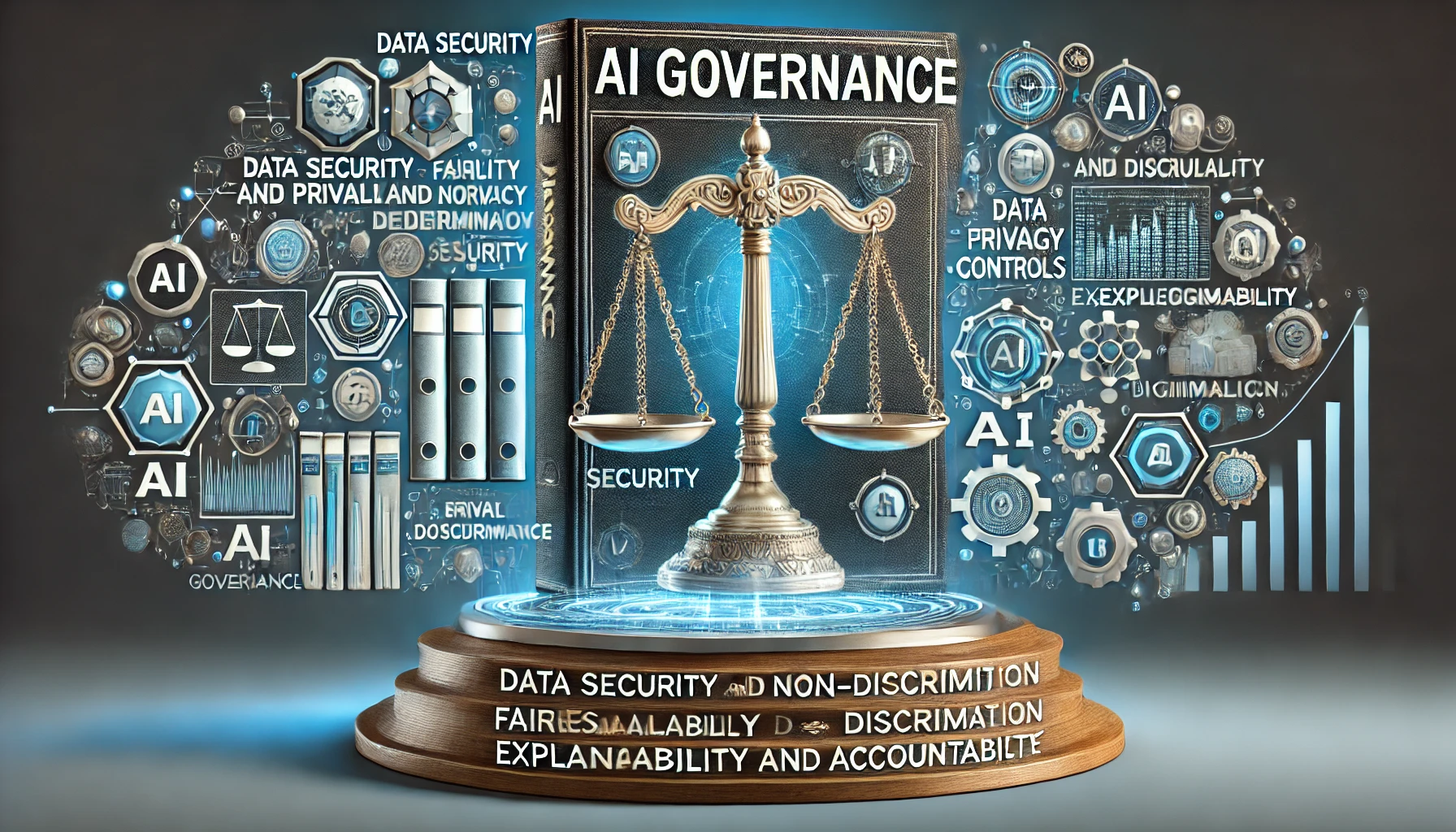
The rapid advancements in AI have resulted in the emergence of AI governance principles and calls for responsible innovation. In order to realize the potential of responsible AI innovation, organizations must be able to translate AI governance requirements into technical controls.
This post reviews the regulatory requirements for AI governance and describes an approach to map them into technical controls, which the organizations can use as a checklist to implement responsible innovation practices. We use an AI application as a working example to illustrate how to apply this approach to enterprise use cases.
AI Governance Regulation
The EU AI Act that came into effect August 1, 2024 is the first of its kind and most comprehensive regulation to address the challenges of AI Governance.
SCOPE
The Act makes a distinction between AI model and AI systems.
- AI Models
- An AI model refers to the algorithm or set of mathematical rules that processes data to make predictions, classifications, or decisions. It is essentially the core component that drives how an AI system operates.
- AI models are developed through techniques like machine learning, statistical analysis, or rule-based logic, where they learn from data to perform specific tasks (e.g., classifying images, predicting financial outcomes).
- AI Systems
- An AI system, as defined by the EU AI Act, is a complete software system that includes one or more AI models and the infrastructure around them. It interacts with its environment, processes inputs, and generates outputs such as predictions, recommendations, or decisions.
- An AI system incorporates an AI model but adds layers of input-output handling, data management, security controls, user interfaces, and compliance features.
AI systems are directly in scope of the Act. If AI model is integrated into a larger application (AI system), it is subject to the rules of the Act. Otherwise its excluded (for e.g., an AI model being used only for R&D).
RISK CATEGORIES
The Act adopts a risk-based classification approach. It identifies four categories of risk:
- Unacceptable risk:
- AI systems that pose a clear threat to the safety, livelihoods, and rights of people
- These include systems such as social scoring by government, real-time surveillance without targeted scope and legal safeguards, and manipulative systems targeting vulnerable age groups (such as children and elderly).
- These systems have an outright ban.
- High risk:
- AI systems that include specific high risk use cases, with significant impact on fundamental rights, safety, or critical societal functions.
- These systems include AI systems used for facial recognition or biometric identification with targeted scope or legal safeguards, such as those used by law enforcement for surveillance purposes, or AI systems used for recruitment, hiring, or employee evaluation.
- They must meet the mandatory risk assessments and conformity assessments under the EU AI Act.
- Limited risk:
- AI systems that pose limited risk to individuals or impact on fundamental rights or safety.
- These systems include chatbots, recommendation systems used on e-commerce sites, or for targeted advertising or automated marketing.
- These systems will carry transparency obligations so users know AI is present.
- Minimal/no risk:
- AI systems that pose no or negligible risk to individuals’ safety or rights.
- These systems include AI-enabled video games, productivity tools like Grammarly, or spam filters.
- They carry no additional compliance obligations.
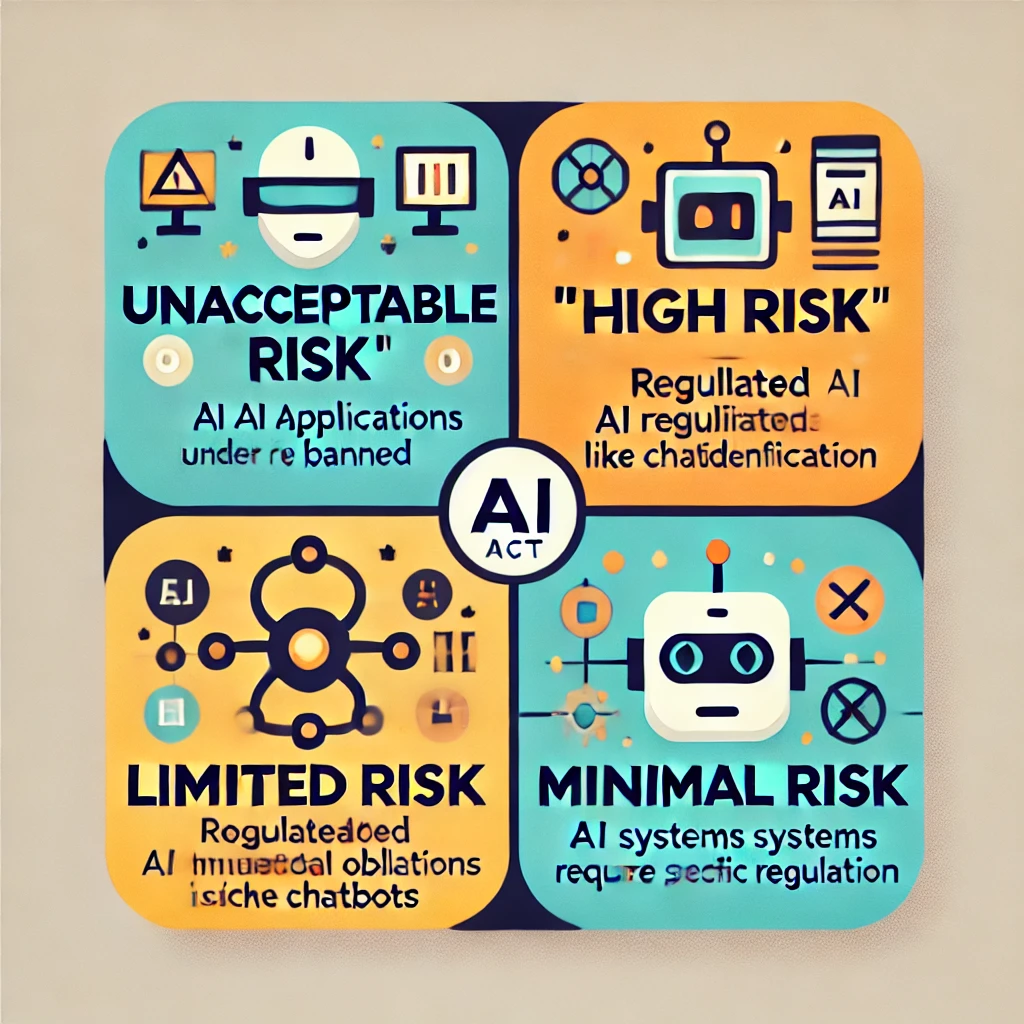
AI Governance Challenges
Under the EU AI Act, there are several requirements related to governance and ethics that need to be addressed when deploying AI systems. They can be categorized at a high level into the challenges depicted in the following chart.
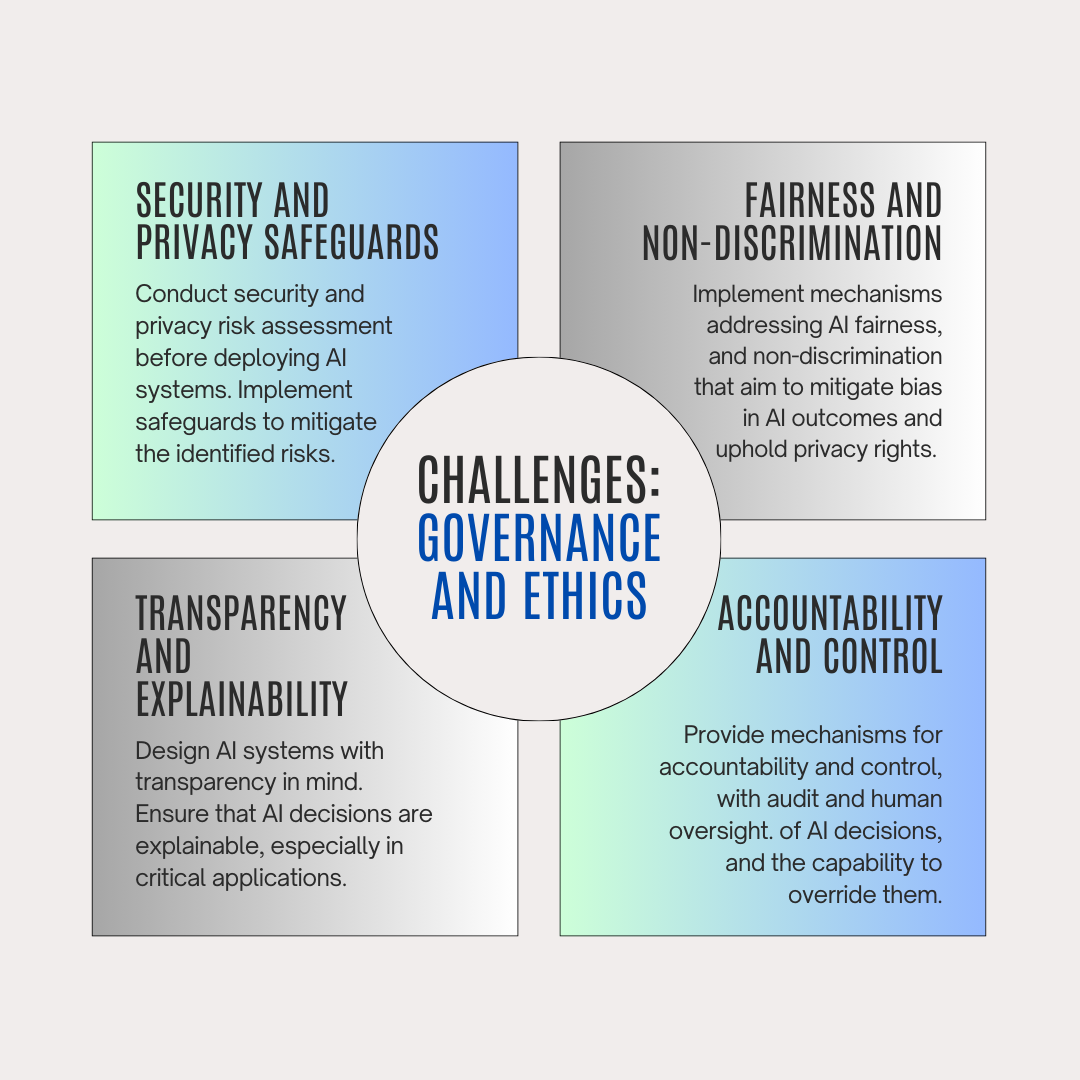
Below, we will discuss these challenges and describe the technical controls that can be used to address them.
#1 Security and Privacy
DATA PRIVACY RISKS
While the EU AI Act doesn't directly regulate data protection, it requires in-scope AI systems to comply with existing data protection laws, including GDPR.
- For limited risk systems under the Act, this means that they continue to respect GDPR principles that apply to processing of personal data, such as obligations related to notice, consent, and data subject rights.
- For AI systems that engage in automated decision-making and hence categorized as high-risk under the Act, this means a stricter application of GDPR, including documentation for Data Privacy Impact Assessments (DPIAs) performed under the GDPR as well as conformity assessments performed under the Act.
DATA SECURITY RISKS
The Act emphasizes the need for AI systems to be developed, deployed, and maintained in a manner that ensures data security in compliance with existing laws.
- For limited risk systems under the Act, this means that they continue to respect GDPR principles that apply to security of personal data, such as obligations related to mitigating the risks associated with data breaches, unauthorized access, manipulation, or misuse of data.
- For AI systems that are categorized as high-risk under the Act, this again means a stricter application of GDPR, including mandatory enforcement of secure by design principles, in addition to risk management system, governance practices, incident response plans, and conformity assessments performed under the Act.
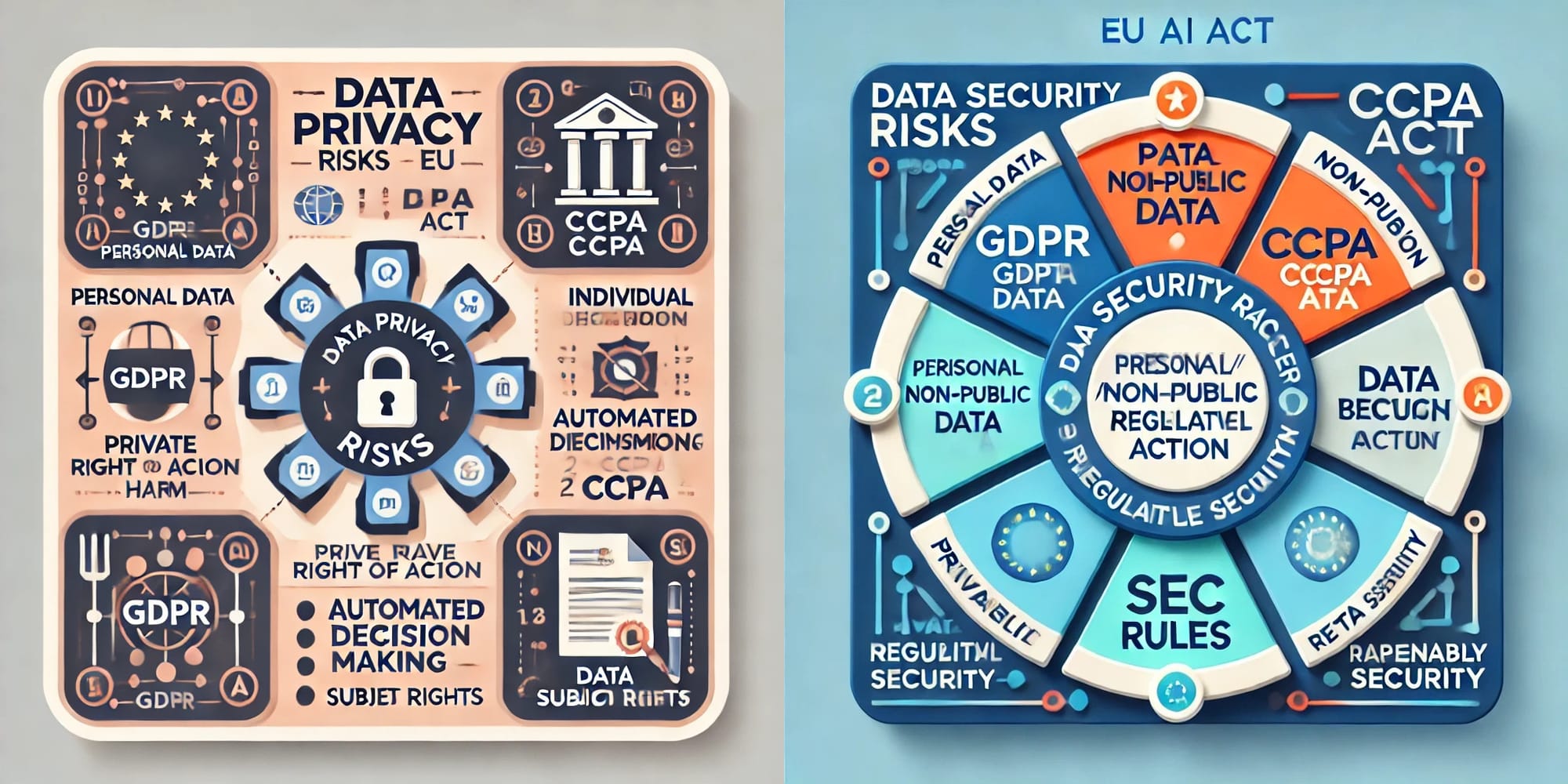
EXAMPLE: SECURITY AND PRIVACY CONTROLS
The following table provides a list of security and privacy controls that apply to Limited and/or High risk only systems. This list can be used for scoping the AI governance efforts for an organization depending on the risk classification level of the AI application being reviewed.
- Limited + High
- High Only*
*This means these requirements are not explicitly called out for non-High risk systems in the EU AI Act. While they may indirectly be implied by GDPR under reasonable security safeguards (for e.g., basic degree of pen-testing, logging and monitoring, and incident response), this mapping is focused on contrasting the explicitly required controls under the EU AI Act for High vs. Limited risk systems.
#2 Fairness and Non-Discrimination
The EU AI Act requires in-scope AI systems, particularly the high-risk systems, to comply with stringent fairness and non-discrimination requirements, including rigorous testing and conformity assessments.
While limited risk systems under the Act are encouraged to avoid bias and discrimination in their design, they are not required to perform rigorous testing or undergo conformity assessments.
EXAMPLE: FAIRNESS AND NON-DISCRIMINATION CONTROLS
#3 Explainability and Transparency (XAI)
The EU AI Act requires in-scope AI systems, particularly the high-risk systems, to comply with stringent explainability and transparency requirements, including detailed documentation and conformity assessments.
On the other hand, limited risk systems under the Act are only required to meet transparency obligations to inform individuals about the use of AI. They are not required to maintain detailed documentation or undergo conformity assessments.
EXAMPLE: EXPLAINABILITY AND TRANSPARENCY (XAI) CONTROLS
#4 Accountability and Control
The EU AI Act requires in-scope AI systems, particularly the high-risk systems, to comply with stringent accountability and control requirements, including ongoing monitoring and conformity assessments.
While limited risk systems under the Act are encouraged to have accountability and human oversight, they are not required to perform ongoing monitoring or undergo conformity assessments.
EXAMPLE: ACCOUNTABILITY CONTROLS
Enterprise AI Use Cases
We will now apply this approach to meet AI governance obligations for an AI application supporting a set of enterprise AI use cases.
Many enterprise AI applications are based on a technique called Retrieval Augmented Generation (RAG). RAG combines information retrieval with natural language generation to create powerful AI systems capable of handling complex tasks.
For the purposes of illustration, we will assume a RAG-based AI system for a healthcare organization that operates in the US and EU. The system can support the following use cases:
- Use Case #1: Clinical Decision Support (CDS) Systems: It can assist in making diagnostic and treatment decisions by retrieving relevant medical literature, patient history, and clinical guidelines, then generating a summary.
- Use Case #2: Health Chatbots and Virtual Assistants: It can provide anonymized responses to medical questions by retrieving relevant content from databases of symptoms, conditions, and treatments.
- Use Case #3: Patient Insurance Data Reporting: It can retrieve patient claim records for insurance purposes to generate comprehensive patient summaries and make future eligibility decisions for medical coverage.
We will further assume that this AI system is both used internally by the organization as well as deployed for external use in other third party systems.
EXERCISE: MAPPING REQUIREMENTS TO CONTROLS
Conclusion
This post provided a review of AI governance regulations and an approach to map AI governance requirements into technical controls. This serves as a checklist for organizations on their journey toward implementing responsible innovation practices.
Connect with us
If you would like to reach out for guidance or provide other input, please do not hesitate to contact us.